Tecton Named Gartner Cool Vendor
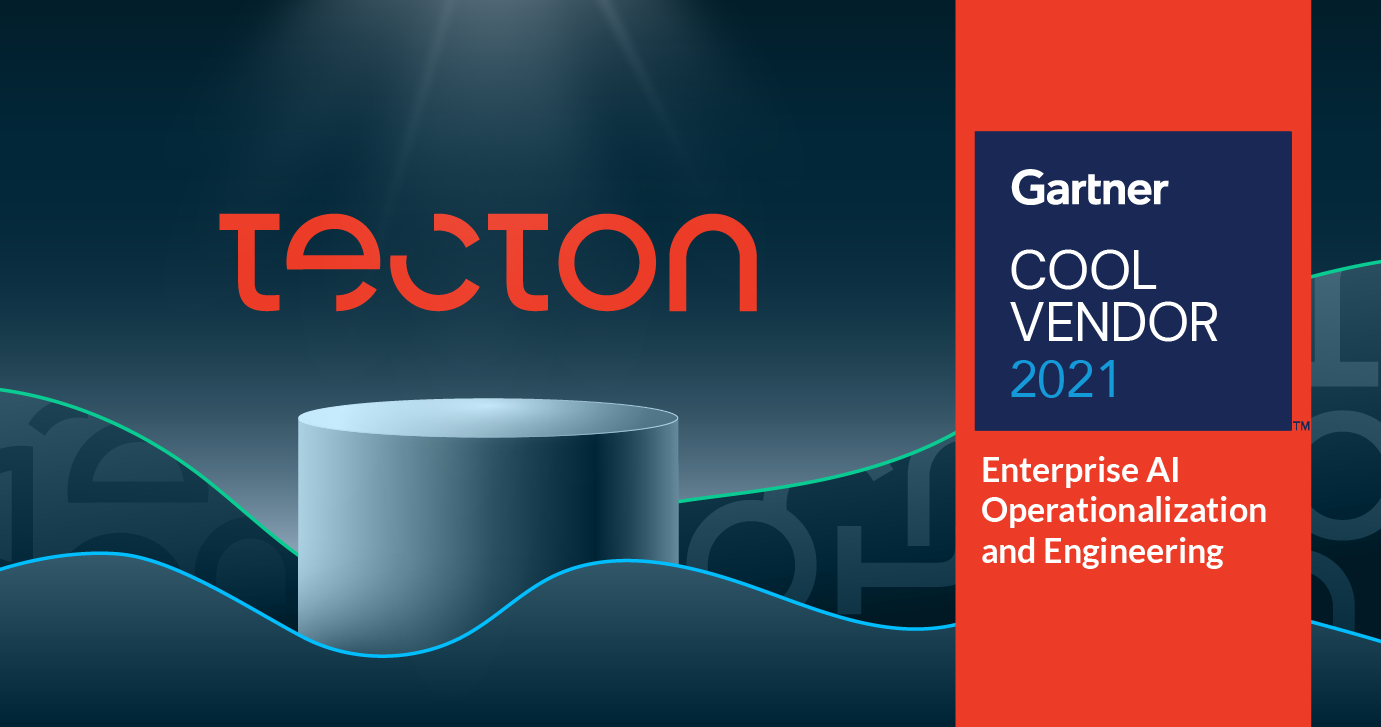
We’re excited to announce that Tecton has been named a 2021 Gartner Cool Vendor in Enterprise AI Operationalization and Engineering(1). This is great recognition for Tecton’s vision of solving the data problem for ML by making feature stores accessible to everyone.
Organizations are increasingly investing in Operational ML (ML used for online, automated decision making) to power a new class of production applications. For example, Uber uses tens of thousands of Operational ML models for driver-rider matching, surge pricing, spatio-temporal supply optimization, and ETA forecasting. Insurance companies are using ML to personalize the underwriting and quoting process. Banking and financial services are relying on real-time ML to improve fraud detection.
But, for all the promise of Operational ML, it is hard to achieve at scale. When building traditional apps, engineering teams really just need to worry about building and deploying code. In the world of Operational ML, enterprises now have to deploy code, models, and data (ML features) to production. In the absence of proper tooling, it often takes many months to get a single model over the finish line, and the ROI on ML projects is often unsatisfactory.
From Gartner’s report: “Tecton provides an enterprise data feature store that enables data scientists to build, reuse and monitor features for batch and real-time analytics through a centralized repository to accelerate machine learning (ML) model development and deliver reliable data to models in production. Rather than just offering a “store”, Tecton provides end-to-end management and orchestration of the feature lifecycle, from feature creation and evaluation, to deployment, sharing, security, governance and monitoring.“
There’s a lot of information in this Gartner statement, and it’s a great description of what we do. Let’s unpack the key points:
What Tecton does: the enterprise feature store. We provide an enterprise feature store that serves as the bridge between your enterprise data and ML models. ML models will only ever be as good as the data that we provide to them. Enterprises have massive amounts of analytic data, but it needs to be ‘operationalized’ to be useful to models. It needs to be refined, served offline for model training, and served online with production SLAs for real-time inference. That’s the role of the feature store.
Read more on “What is a feature store?”.
Why do we need it? We believe that feature stores are an essential part of the modern MLOps stack. Without a feature store, ML teams spend months engineering features, generating training datasets, and building production data pipelines. A feature store enables data scientists to quickly and reliably build, use, share, and monitor ML features. This in turn allows ML teams to get models to production faster, while increasing prediction accuracy with better data.
Read how Tide and Atlassian use the Tecton feature store.
How is Tecton unique? Despite the feature store name, Tecton is more than just a ‘store’. Tecton manages the entire feature lifecycle, from development to production. More specifically, Tecton:
- Provides a declarative framework for defining features as code, and manages feature definitions in a Git-backed repository
- Automates feature transformations to materialize both historical data and fresh data
- Allows data scientists to search, discover, share and re-use features across models
- Generates accurate training datasets with row-level time travel
- Serves data online with production service levels for real-time inference
- Monitors features for service levels and data quality
The Gartner Cool Vendor recognition is an important milestone both for Tecton and the development of the feature store category. It acknowledges the importance of feature stores to bridge the gap between enterprise data and ML models, and the unique solution that Tecton has built to manage the complete lifecycle of ML features.
We’re excited to continue helping all organizations solve some of their greatest challenges in operationalizing ML. Please reach out if you’d like to see a demo of Tecton by signing up for a free evaluation or emailing us at info@tecton.ai.
If you are a Gartner subscriber, you can read the full report here.
Resources:
(1) Gartner, “Cool Vendors in Enterprise AI Operationalization and Engineering”, Chirag Dekate, Farhan Choudhary, Soyeb Barot, Erick Brethenoux, Arun Chandrasekaran, Robert Thanaraj, Georgia O’Callaghan, 27 April 2021 (report available to Gartner subscribers here: https://www.gartner.com/doc/4001037)